Hauptinhalt
Topinformationen
Yusuf Brima
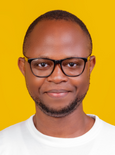
Room 50/228
Phone +49 (0)541 969-3371
Institute of Cognitive Science,
Wachsbleiche 27,
49090 Osnabrück, Germany
Supervisors
At the Interface of Signalling Theory and Deep Learning
Deep Learning has had a groundswell of successes in domain-specific tasks in the recent past primarily due to the availability of larger datasets and massive compute power. In contrast, humans (and other intelligent beings), are able to learn statistical regularities and world models through intuitive physics, that capture the disentangled causal structures of physical and abstract worlds. For example, a child learns motion, gravity, object permanence and other intuitive notions like object categorization, causal mechanisms at a very early age. This is achieved through their ability to learn disentangled semantic causal representations through attentional mechanisms.
One of the primary domains where these levels of hierarchical representations are inherent is language – a basis for fusion-fission systems , which is very algebraic and statistical in nature due to its compositionality. Language is one of the pinnacles of human cultural artefacts as it allows for remarkably complex flow of information. It intermediates cooperation – an evolutionary high payoff fitness strategy, as it is a primary evolutionary stable strategy signalling mechanism that has high bandwidth information transmission at minimal cost to the signaller.
My work, thus, focuses on bridging the comparative study of signalling via vocalizations using principles of deep representation learning to understand latent factors of variation that underpin signalling mechanisms in communicative processes under selective pressures. That will help lay the foundation in understanding cognitive processes that underlie non-human proto-language communication: context, meaning and effect.
Research interests:
• Representation Learning
• Computer Vision
• Causal Inference
• Explainable and Fair AI
• Mathematical Modelling
• Computational Entrepreneurship
Topinformationen
Publications
Brima Y, Krumnack U, Pika S, Heidemann G. (2024) Understanding Self-Supervised Learning of Speech Representation via Invariance and Redundancy Reduction. Information 15(2):114.
DOI: https://doi.org/10.3390/info15020114
Brima, Y., Atemkeng, M., Tankio Djiokap, S., Ebiele, J., & Tchakounté, F. (2021).
Transfer Learning for the Detection and Diagnosis of Types of Pneumonia including Pneumonia Induced by COVID-19 from Chest X-ray Images. Diagnostics, 11(8), 1480.
DOI: doi.org/10.3390/diagnostics11081480 (not RTG related)
Preprints
Brima, Y., Tushar, M.H.K., Kabir, U. and Islam, T., 2021.
Deep Transfer Learning for Brain Magnetic Resonance Image Multi-class Classification. arXiv preprint arXiv:2106.07333.
DOI: arxiv.org/abs/2106.07333 (not RTG related)